From Data to Action: How Analytics are Shaping the Fight Against Drug and Alcohol Misuse in the US
From Data to Action: How Analytics are Shaping the Fight Against Drug and Alcohol Misuse in the US
The battle against drug and alcohol misuse in the US is complex and multifaceted. With a diverse population, varying social determinants of health, and a constantly evolving landscape of substances and trends, crafting effective prevention and intervention strategies requires a nuanced approach.
Fortunately, in this data-driven age, powerful tools have emerged to help us navigate this complex terrain. Data analysis is no longer just crunching numbers; it’s becoming a vital weapon in the fight against addiction, informing prevention, intervention, and resource allocation with unprecedented precision.
Data Delving: Unveiling Patterns and Predictions
Data Delving: Unveiling Patterns and Predictions
Traditional methods of prevention and intervention often relied on broad-brush approaches, targeting entire communities or demographics. However, data analysis allows us to drill down deeper, uncovering hidden patterns and predicting where and how addiction is most likely to take root. This starts with geospatial mapping, which pinpoints areas experiencing high rates of substance use disorder (SUD). For example, the CDC’s National Drug Early Warning System (NDEWS) tracks emergency department visits and overdose deaths linked to specific drugs, providing a real-time picture of emerging hotspots.
But data analysis goes beyond mere mapping. Social media monitoring can identify communities where discussions about drug use are prevalent, allowing for targeted outreach and education campaigns. Social network analysis can reveal connections between individuals, helping to identify high-risk groups for early intervention. And predictive modeling can analyze historical data to forecast future trends and allocate resources accordingly.
Case Study: Stopping Fentanyl in its Tracks
The opioid crisis, fueled by the rise of fentanyl, has ravaged communities across the US. In New York City, data analysis proved crucial in curbing the epidemic. By analyzing overdose data and crime reports, the city identified specific neighborhoods with high fentanyl-related deaths. This information informed targeted police patrols, sting operations, and awareness campaigns in these areas, leading to a significant decrease in overdose fatalities.
Beyond Prevention:
Data-Driven Intervention
Data analysis isn’t just about stopping addiction before it starts; it’s also about helping those already struggling. Electronic health records (EHRs) can be analyzed to identify patients at risk of developing SUD, allowing for early intervention and referral to treatment programs. Machine learning algorithms can analyze patient data to predict treatment success rates, helping healthcare providers tailor individualized care plans.
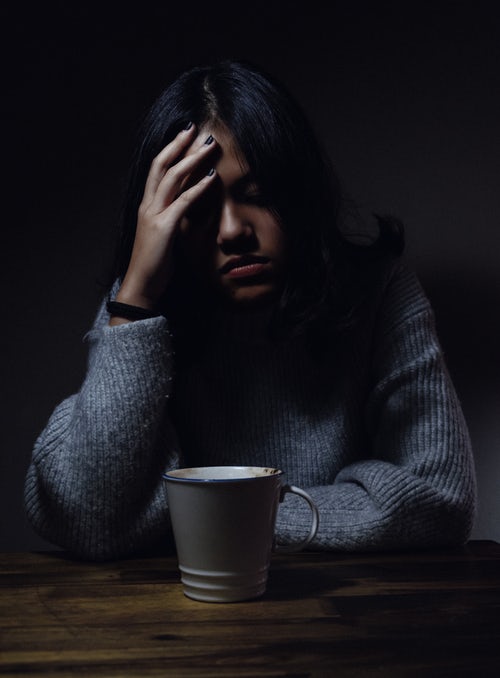
Case Study: Tailoring Treatment for Opioid Use Disorder
One initiative in Massachusetts uses data analysis to personalize treatment for opioid use disorder. By analyzing patient data, including demographics, medical history, and response to treatment, the program can predict which medication and therapy combination is most likely to be successful for each individual. This data-driven approach has led to significantly higher treatment completion rates and improved long-term outcomes for patients.
Additional Case Studies:
Additional Use Cases of Data-Driven Insights
By harnessing these resources through sophisticated analytics tools, researchers and public health officials are gaining unprecedented insights into the complex patterns of drug and alcohol misuse:
Challenges and Considerations
While data analysis holds immense promise, it’s important to acknowledge its limitations. Issues like data privacy, ethical considerations, and potential for bias must be carefully addressed. Additionally, data analysis alone cannot solve the complex problem of addiction. It must be combined with strong public health infrastructure, accessible treatment options, and ongoing community support.
The Future of Data-Driven Solutions
Despite the challenges, the potential of data analysis in the fight against drug and alcohol misuse is undeniable. As technology advances and data collection becomes more sophisticated, we can expect even more powerful tools to emerge. Imagine a future where real-time data analysis informs immediate interventions, where personalized treatment plans are crafted with AI precision, and where targeted outreach prevents addiction before it takes hold. This is the future that data promises, a future where we can finally turn the tide on the addiction epidemic and build healthier communities for all.
Data Sources:
- Centers for Disease Control and Prevention (CDC) National Drug Early Warning System (NDEWS)
- National Institute on Drug Abuse (NIDA)
- Substance Abuse and Mental Health Services Administration (SAMHSA)
- American Journal of Public Health
- Health Affairs